Machine Learning for stainless steel selection on corrosion resistance
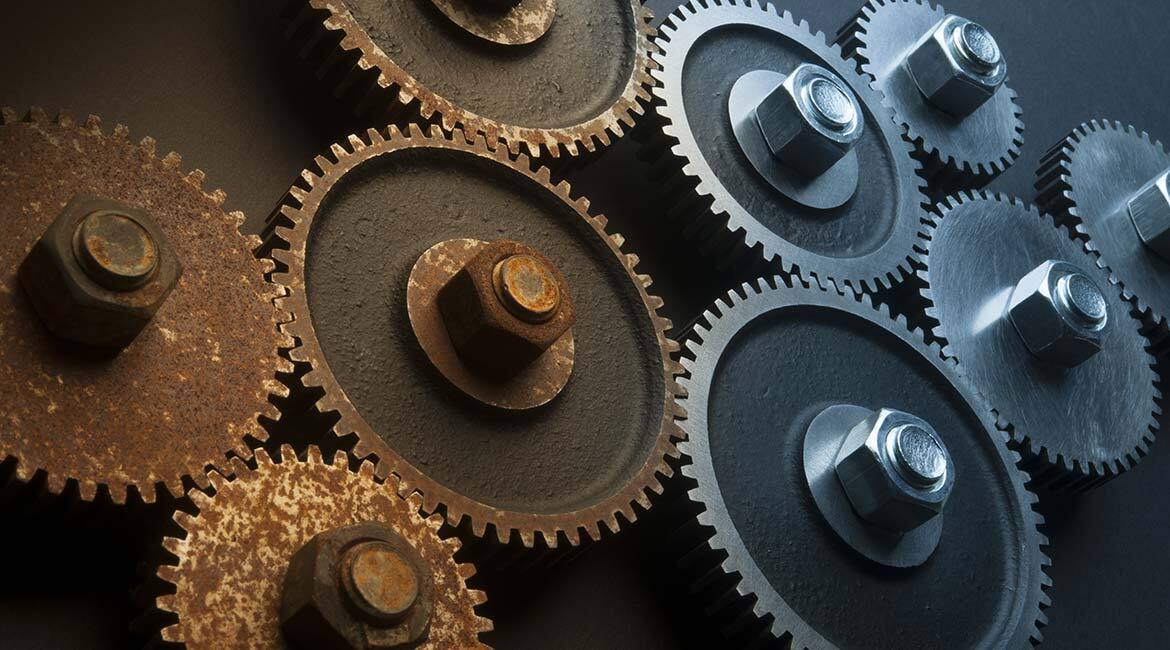
Purchased on Gettyimages.com. Copyright.
This study addresses the vital need for predicting corrosion behavior under various environmental conditions to prevent industrial failures. It explores supervised machine learning techniques to predict this behavior, focusing on stainless steel grades in various corrosive environments, including acids, bases and salts. Traditional experimental methods often struggle to identify key factors in the corrosion process due to its complex, multivariate, and nonlinear nature. The study presents and evaluates multiple machine learning models, including decision tree, support vector machine, random forest, and bagging classifier. Keywords: material selection; corrosion; stainless steel; machine learning.
Choosing the Right Stainless Steel
In recent years, stainless steels (SSs) have taken an important part in various industries because of their useful properties, especially their ability to resist corrosion [1]. When designing structures for industrial use, it is essential to choose the right type of SS, considering both its properties and the environment it will face, since the environment determines the rate at which SS corrodes [2]. Machine learning (ML) is increasingly used in materials research to predict how SS will corrode. Indeed, this can save time and money compared with traditional testing methods [3–6]. The present contribution outlines the development of an ML model enabling users to predict the corrosion behavior of particular SS materials in defined environmental conditions. This model facilitates well-informed material selection across various applications, eliminating the need for costly and time-consuming experiments. The study relies on a dataset containing information about the chemical composition of 34 distinct SS grades, along with details on the corrosive environments’ type, concentration, and temperature. By associating these features with corrosion behavior labels defined by corrosion rates, this research employs various ML techniques and compares their accuracies. Additionally, it assesses how feature creation impacts model accuracy, generating a new dataset in the feature creation phase by substituting electrolyte types with critical ions predominantly contributing to the corrosion reaction. Furthermore, the study endeavors to utilize ML techniques in exploring the relative importance of features in chemical composition and the type of corrosive environment concerning the corrosion behavior of SS alloys. The insights from this analysis can significantly support decision-making in selecting materials for specific corrosive environments.
Overview of the Classifying Method
Figure 1 provides a visual representation of the process used to assess the corrosion behavior of SSs in various corrosive environments. The initial stage involves preprocessing the data before applying it to the ML modeling phase. Two separate datasets, Dataset No. 1 and Dataset No. 2, serve as inputs to the ML models. Dataset No. 1 includes features such as electrolyte type and concentration. By contrast, Dataset No. 2 is a modified version of Dataset No. 1, where electrolyte type and concentration are transformed into the concentration of crucial ions involved in corrosion reactions.

Figure 1 Schematic overview of the processes developed and applied (iterative) to classify and predict corrosion behavior.
Process for Selecting the Right Stainless Steel
As previously mentioned, the main objective of this research is to introduce a useful ML model that helps select the right SS materials for various environments. Figure 2 provides a practical step-by-step diagram outlining the systematic process of selecting the most suitable SS grade based on its resistance to corrosion. Based on the available information about the material (such as its chemical composition) and environmental conditions (type of solution, concentration and temperature), three models have been developed to predict the corrosion behavior of SSs. The choice of these models (Bagging, DT, DT + feature reduction) was made after conducting two comprehensive analyses, taking into account both their accuracy and their practical applicability.

Figure 2 Flowchart illustrating the selection process for SS material using the developed models.
Accuracy Based on Machine Learning Models
Results and analysis revealed the following:
– The Bagging classifier achieved the highest testing accuracy, reaching 94.4% for Dataset No. 1, making it the most effective model for predicting SS corrosion behavior.
– We used a confusion matrix analysis, which goes beyond accuracy, and found that the Bagging model demonstrated the highest precision in detecting “severe” and “poor” corrosion behaviors, which are crucial for accurate identification.
– For Dataset No. 2, the Decision Tree (DT) model achieved the highest accuracy, at 93.53% among the classification models, and was also selected as the most suitable model based on the confusion matrix analysis.
– A feature importance analysis conducted on Dataset No. 2 identified the most influential factors affecting SS corrosion behavior in different environments. These findings offer valuable insights for material selection and design, highlighting hydrogen and sulphide concentrations in corrosive environments and the quantity of alloying elements as key factors, contributing up to 77.8% to corrosion behavior.
Conclusion
The developed model can predict the corrosion behavior of various SS grades in 14 different corrosive environments (Dataset No. 1) without the need for experimental testing. To enhance the model’s applicability to a broader range of environments, we created a second model (Dataset No. 2) that considers only the concentration of four critical corrosion ions, which allows us to predict corrosion behavior with less environmental information. These models have the potential to prevent corrosion-related failures by providing essential information on SS behavior in specific environments. Predicting corrosion behavior without experimental testing saves time and resources, while improving the reliability of corrosion-related decisions. Future work could involve expanding the dataset to include more environmental conditions and other types of steels beyond SS in our corrosion prediction model.